

The \(R^2\) changes computed using anova() results correspond to differences in \(R^2\)s in lm() results for each model: 0.1311 – 0.02855 = 0.10255 for Model 2 and 0.1969 – 0.1311 = 0.0658 for Model 3 (with rounding errors). Residual standard error: 1.427 on 95 degrees of freedom Residual standard error: 1.476 on 96 degrees of freedom Residual standard error: 1.553 on 97 degrees of freedom Summary() of an lm object shows coefficients of variables: By adding pets, the model accounts for additional \(SS\) 15.846 and it was statistically significant again.
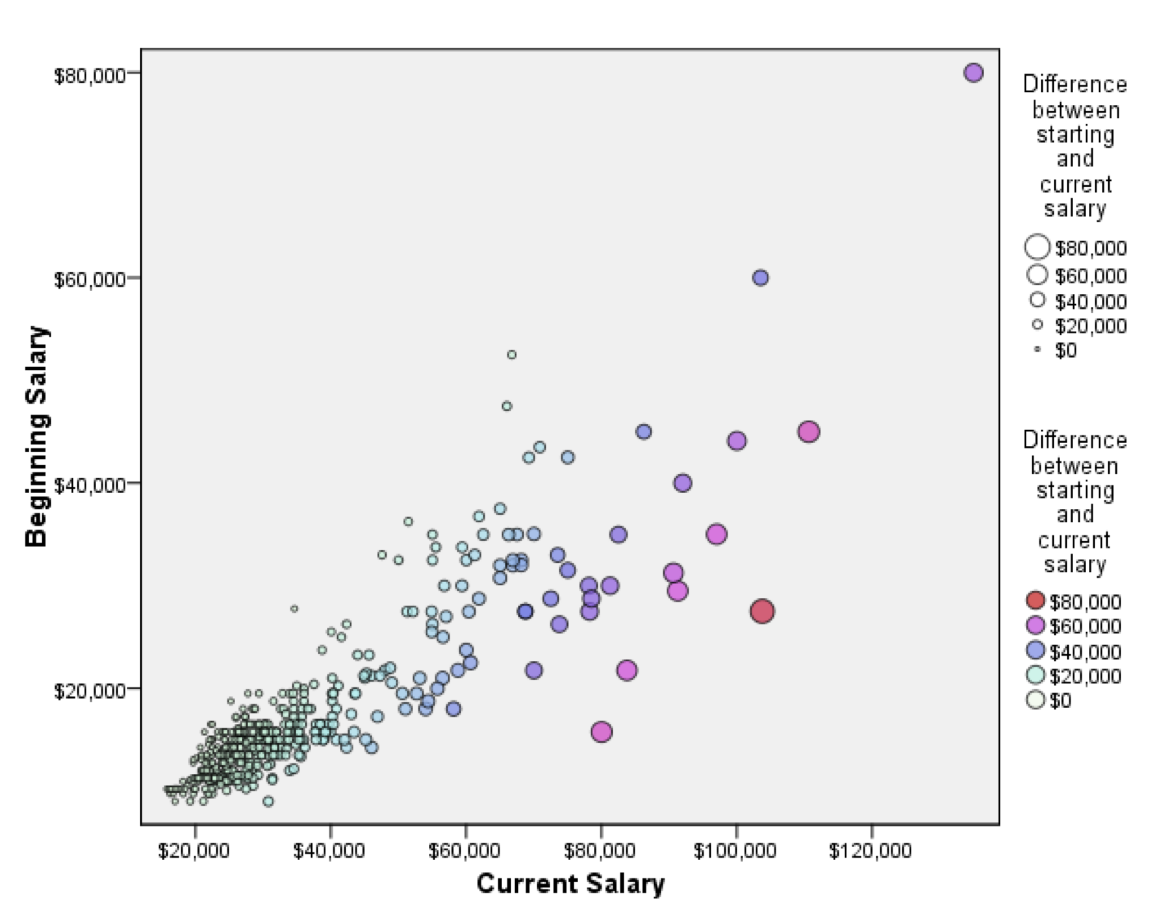
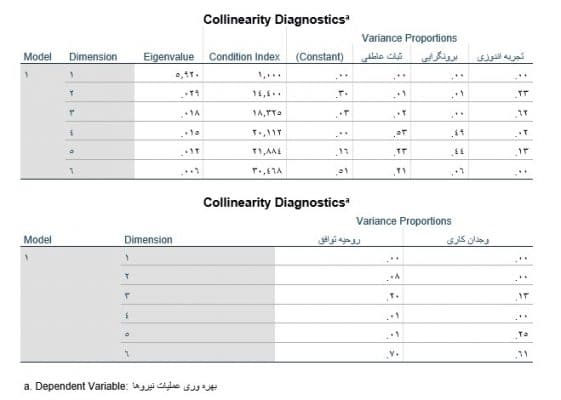
If so, we can say that the number of pets explains an additional 6% of the variance in happiness and it is statistically significant. In this example, we’d like to know if the increased \(R^2\). If the difference of \(R^2\) between Model 2 and 3 is statistically significant, we can say the added variables in Model 3 explain the DV above and beyond the variables in Model 2. Our interest is whether Model 3 explains the DV better than Model 2. Model 3: Happiness = Intercept + Age + Gender + # of friends + # of pets (\(R^2\) =. Model 2: Happiness = Intercept + Age + Gender + # of friends (\(R^2\) =. Model 1: Happiness = Intercept + Age + Gender (\(R^2\) =. In the following step (Model 3), we could add the variables that we’re interested in. Here we would replicate previous research in this subject matter. In the next step (Model 2), we could add known important variables in this line of research. The first model (Model 1) typically includes demographic information such as age, gender, ethnicity, and education. However, we’d like to investigate if the number of pets could be an important predictor for happiness. In this line of research, the number of friends has been a known predictor in addition to demographic characteristics. Let’s say we’re interested in the relationships of social interaction and happiness. In many cases, our interest is to determine whether newly added variables show a significant improvement in \(R^2\) (the proportion of explained variance in DV by the model). In this framework, you build several regression models by adding variables to a previous model at each step later models always include smaller models in previous steps. This is a framework for model comparison rather than a statistical method. Hierarchical regression is a way to show if variables of your interest explain a statistically significant amount of variance in your Dependent Variable (DV) after accounting for all other variables.

When do I want to perform hierarchical regression analysis? The hierarchical regression is model comparison of nested regression models. This post is NOT about Hierarchical Linear Modeling (HLM multilevel modeling).
